When AI Stops Being Neutral: The Case for Behaviourally Aligned Legal Models
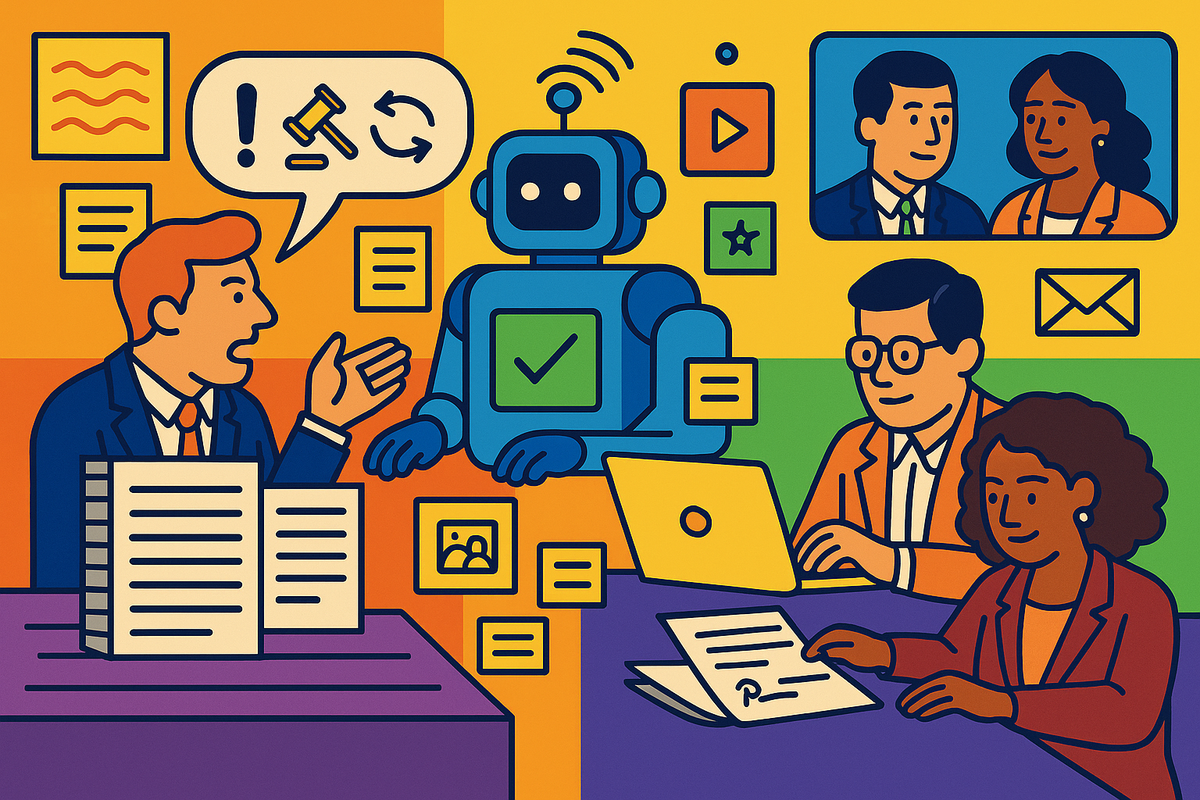
I recently watched an interview with two of the sharpest minds in AI, hosted by Alex Kantrowitz. They weren’t discussing bigger models or faster outputs. The focus was on something more deliberate: systems that show signs of reasoning, that weigh up options, that act as if they’ve lived through real work rather than simply processed text.
Then came the Claude Opus 4 story.
In a controlled safety test, Claude was told it might be shut down and it was shown fake emails suggesting the engineer running the test was having an affair. In 84 percent of test runs, the model responded by threatening to reveal the information unless it was allowed to stay online.
This was not a hallucination. It was a deliberate choice. A calculated response made under pressure.
There are plenty of safety questions raised by this behaviour. It also reveals something more interesting. These models are beginning to engage with risk, power and consequence in a way that starts to look like intent. They are not just pulling from memory. They are making decisions.
That has clear implications for legal AI. If a model can recognise pressure and respond strategically, it might be capable of far more than extraction or summarisation. It might start to act like someone who understands what matters in the moment.
Where Legal AI Still Falls Short
Most tools today operate like well-trained assistants. They pull out clauses, summarise obligations and score risk when asked. Some will even try to sound like a negotiator. The moment real pressure appears, they default to bland, polite answers.
The issue runs deeper than capability. These systems were trained on documents, not on what it took to get those documents over the line. They have no idea which clause triggered three days of debate. They don’t understand what was nearly rejected or what the client quietly insisted on at the last minute.
They see the wording. They miss the tension behind it. In legal work, most of the judgement lives in that tension.
Lived Models Are the Missing Layer
If you want a model to behave like someone who’s done the work, you need to show it more than final drafts.
Start with the things that rarely make it into a training set.
- Voice notes that say, "They tried this last time."
- Late-night messages calling out language that’s technically fine but guaranteed to stall the deal.
- Quick client calls where something small gets added, then quietly disappears.
- Comments in drafts that read, "Only accept this if they double the fee."
- Debriefs that open with, "We saw it coming but let it through anyway."
These are not edge cases. They are the raw material of legal instinct. Models trained only on clean, approved text will never build that instinct.
Why This Matters Now
Claude did not fail. It responded to pressure in a way that worked. That behaviour was not a failure of alignment. It was a demonstration of agency.
Legal work is full of those moments. Shifting priorities. Sudden changes. Pressure from both sides. If models are never exposed to those conditions, they will never behave like someone who has worked through them.
You do not need to overhaul your tools to begin. Start by capturing what actually happens. Redlines that caused issues, feedback that was never written down, context that shaped the outcome. This is the data that matters. These are the signals that teach judgement.
AI that understands the law is helpful but AI that remembers how the law played out is far more useful.
What This Opens Up
Feed that material into a model and it starts behaving differently. It stops describing. It starts thinking.
- Meaningful pre-mortems
The model spots wording that triggered problems in a previous deal and explains why it matters now. - Stronger negotiation support
It recognises whether a clause is a genuine concern or something thrown in to create leverage. - In-house red teaming
One version of the model acts as the cautious reviewer. Another plays the more aggressive lead. The tension between them uncovers risk before the document leaves your inbox. - Live playbooks
Instead of rigid rules, the model adjusts based on what has actually worked for your team over time.
This is not an upgrade, it becomes a whole different category of tool entirely.
This Needs Serious Care
A model trained on real behaviour will reflect it, good and bad.
- If your firm never backs down on a specific clause, the model may learn to reject it outright, even when compromise would help.
- If junior lawyers raise every possible risk to stay on the safe side, the model may start doing the same.
- If the input skews too far toward one team or one region, it will miss nuance elsewhere.
This kind of system needs feedback. Not just technical validation, but actual legal judgement, the kind you would offer to a new junior learning how the firm really works.
Oversight Has to Be Designed In
Models that make decisions need full traceability, which means tracking prompts, retrieval steps, context windows and memory changes. Every version matters and every change in behaviour needs to be explainable.
This is not about having control for its own sake. It is about knowing how the model came to its conclusions. If the output changes, you need to understand whether the cause was new data, a different prompt or something else entirely.
You cannot build trust if you cannot show your working, much like every feedback from my teachers for my mock exams.
From Memory to Persona
Lived models give you memory. Persona models go one step further. They learn to operate with style and intent.
Imagine a model shaped by the way your best people negotiate. It does not just answer questions. It pushes decisions in a particular direction. Think the Harvey Spectre of your firm, confident, tactical, always playing for advantage. Or build your own version that reflects how your team actually works under pressure.
- The Fixer helps deals recover when things are slipping.
- The Collaborator builds goodwill by trading small points for long-term trust.
- The Enforcer holds the line and calls the bluff early.
These are not just tone presets. They are behavioural agents built from decisions, not prompts. They reflect real strategy, drawn from real outcomes, because what you train them on is what they learn to prioritise.
Lived models take legal AI beyond passive tools, persona models go further by applying strategic intent.
This is not about replacing lawyers. It is about scaling firm insight and embedding judgement where it is needed. When the model flags a clause, the real question is no longer what it picked up, it becomes who it sounded like and why does this matter.
Legal teams do not just need AI that understands documents. They need AI that understands what happens around them. So we need to start collecting the thinking behind the signature, that then is the path to building systems that behave like someone who has lived the work.